AI has a ‘social impact’ warns Apple’s Carlos Guestrin
I’ve just spent an incredibly interesting half-hour listening to Apple’s senior director of machine learning and Artificial Intelligence, Carlos Guestrin, who spoke at Geekwire’s CloudTech Summit last week. I think you need to know more about what he said.
Smart machines need smart data
Guestrin, who is also the Amazon Professor of Machine Learning at the University of Washington, spoke about how AI is making a huge difference every day of our lives, but has a history far beyond the current trope.
To illustrate this, he pointed to Frank Rosenblatt’s 1957 Perceptron experiment in which a machine was taught to predict when a shape would appear.
While the shift from rule-based to machine intelligence has enabled much more innovation, there is a danger in trusting data too much.
To illustrate this danger, Guestrin glanced at the history of digital photography and the infamous ‘Shirley’ cardsused by photo processing companies to get the exposure right when printing pictures.
Built-In stupid…
“The choice of data implicitly defines the user experience”, he explained.
All those Shirley cards depicted light-skinned women. This meant that exposure for pictures of light skinned people ended up being qualitatively better exposed than those of darker people – you can see this difference in old photos. It’s only in the 90’s that this systemic prejudice began to erode.
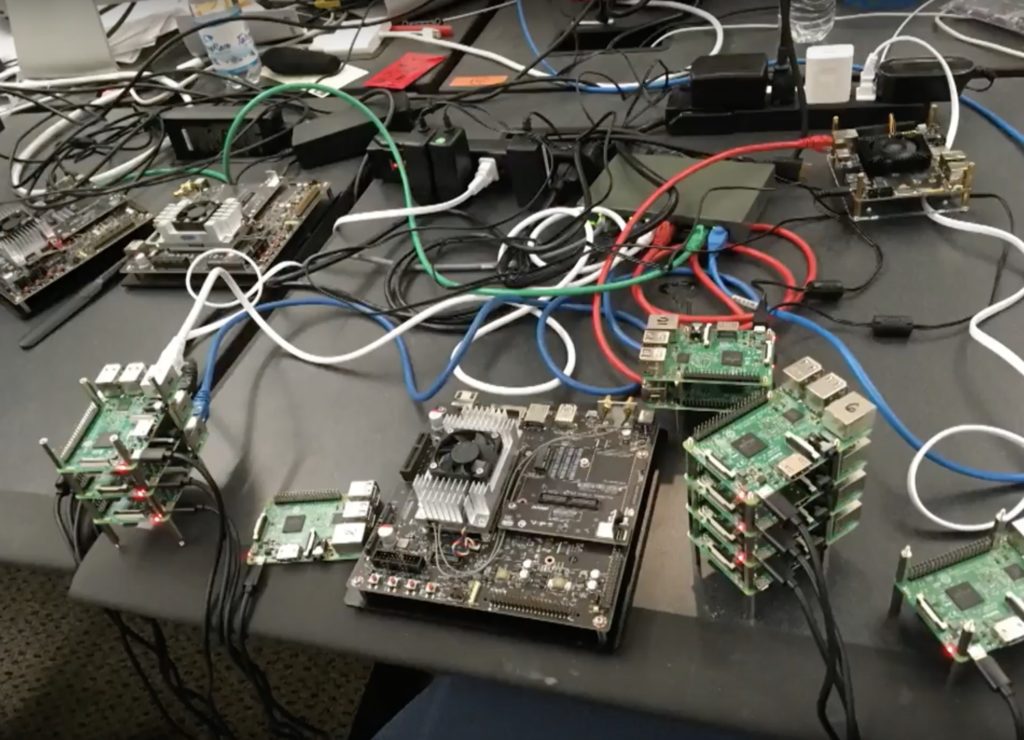
Is this one of Apple’s AI research labs?
“Many studies have shown that if you just train an ML system from data that is randomly selected from the Web, you end up with a system that is racist, misogynist, and sexist, and that’s just a mirror to our society.
In saying this he is addressing my greatest fear around AI: that in a society more or less dominated by fat, straight, prejudiced, white, rich men and those who work for them, AI will end up formalising their prejudice within its thinking.
“So, it’s not enough just to think about the data that we use but also how that data reflects our culture and values that we aspire to,” he said. “There is now a trend not just to thinking about the business metrics but the social impacts machine learning will have.”
Four trends to watch
Guestrin talked about four trends that will influence the future of AI and machine learning development. I’ve summarized them here, but they may be inaccurate or inferences missed, so I really recommend listening to Guestrin yourself:
Trend #1: The shift to high-performance computing type workflows
He mentioned an example in which LIDAr data needs more intensive computational workflows, the kind of deep learning enabled by High performance computing This is used for speech recognition systems, emotional analysis and so on.
Trend #2: More specialised hardware
A lot more specialised hardware, such as Apple’s A11 chip which includes a neural engine with special silicon dedicated to machine learning models.
Trend #3: More hardware targets
There are more hardware targets, more deep learning frameworks and the need to figure out how to apply all these to each piece of hardware. He mentioned the TVM end to end optimization stack for deep learning. This allows you to easily connect machine learning libraries with a wide range of hardware targets by offering an optimization stack that enables this. Guestrin claimed TVM could beat custom solutions for this task.
Trend #4: Simplicity of adoption of machine learning systems
Training deep learning systems is challenging. He expects a move to the commoditization of pre-trained machine learning models (Apple offers Apple Vision tools to developers, for example).
For specific problems using specific data you need to create your own solutions.
Citing an example in which a developer build a “dog detector” using a few lines of code, he explained Apple’s move to build task-focused pre-built libraries that you can use to load and train models for AI (Create ML). The approach means you can “empower the creativity of developers,” he said.
He showed this ARKit2-based Sudoku-solving app as an example of this.
Inclusivity
He explained why it matters that people understand how the AI makes decisions. A doctor making life or death decisions will need to understand how the machine made its decision in order to ascertain if the right decision was reached – that’s why it is important these solutions are easy to understand, even if what they do is exceedingly powerful.
The implication is that AI can’t just be prescriptive, it must also explain why it makes its recommendation — it may be good at data analysis, but a human may still have better insight.
Providing more transparency and “explainability” can help us build better models, he said.
(Interesting, he pointed to an example of an AI that can identify a Stop sign).
He stressed how brittle AI can be – a small change in input (such as something as simple as an extra question mark) can make a big difference to accuracy.
It’s a fascinating talk with relevance to the future of how we live.
Smart technologies are already in use worldwide. AI is already making decisions that impact your daily life — think about traffic management in cities worldwide, or energy supply. These technologies are profound, already impact you, and you should understand what’s happening. I think this is an important talk. Please pass it on.